Unlocking the Secrets of Artificial General Intelligence: Learning Like a Child
Imagine training an AI as akin to raising a child. This enlightening perspective is championed by renowned Christopher Kanan, an associate professor in the Department of Computer Science at the University of Rochester. Kanan advocates an innovative approach, borrowing insights from neuroscience and child development to cultivate AI systems that learn and adapt through exploration, curiosity, and positive reinforcement.
“Many issues with current AI algorithms may be solved by integrating principles from neuroscience and child development,” Kanan asserts. This intriguing analogy challenges us to rethink how we design AI, emphasizing that advancements must come with adequate guardrails. Late-stage corrections might create a scenario where a mishandled AI could become a dangerous entity.
Insights from Christopher Kanan: A Q&A
What is Artificial General Intelligence (AGI) and How is it Different?
- AGI seeks to develop systems that understand, reason, and learn like humans, far surpassing current artificial narrow intelligence (ANI).
Kanan: Traditional AI focuses on task-specific systems, known as ANI, which excel in areas like image recognition and strategic game playing. These systems can outperform humans in their defined tasks but lack the broader cognitive abilities found in humans.
By contrast, AGI aims to create versatile systems capable of comprehending and adapting to a multitude of tasks. Although AGI remains an aspiration, the journey toward it is ongoing. The concept of artificial superintelligence (ASI), which would vastly exceed human capabilities, still resides in the realm of speculation and science fiction. My lab seeks to draw parallels with natural learning processes to edge closer to achieving AGI, allowing machines to adapt much like children do.
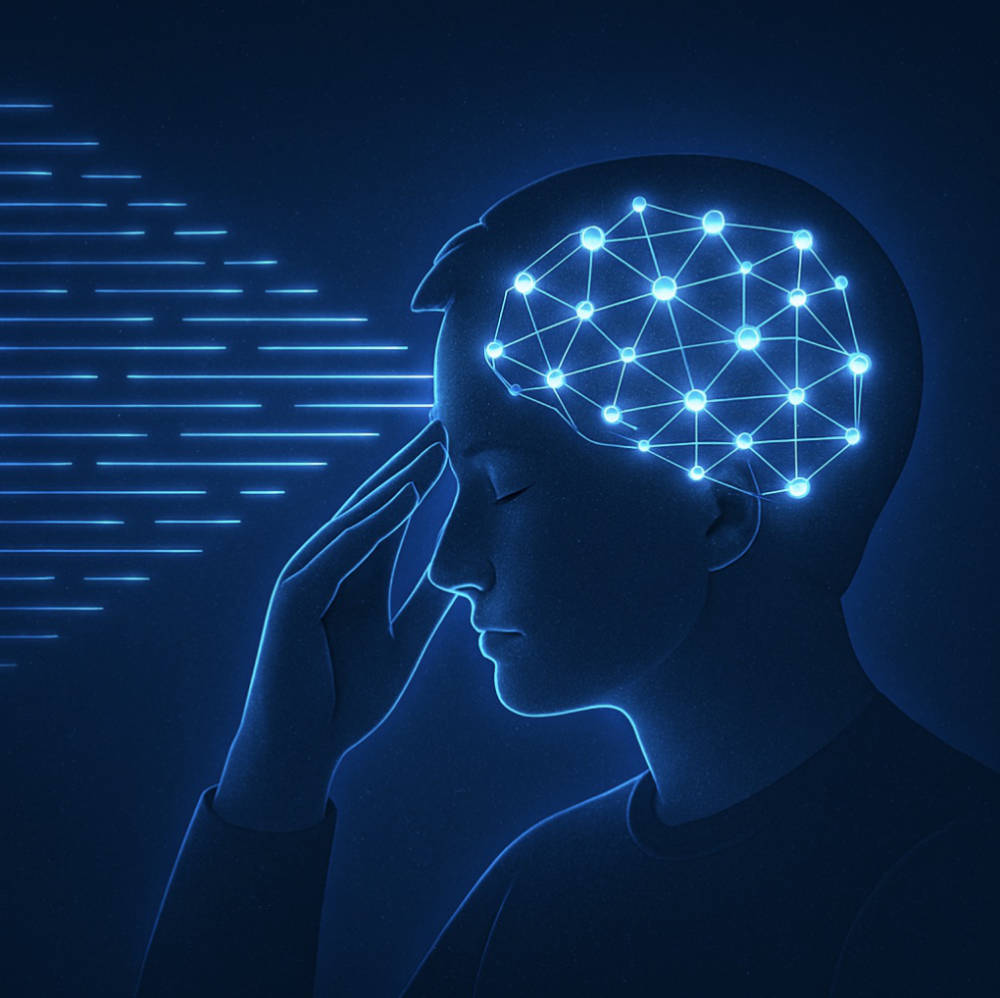
How Does AI Learn?
- Deep neural networks equipped with extensive data inform AI learning.
Kanan: The success of ANI is primarily attributed to deep learning—a method that utilizes vast amounts of human-annotated data to train AI systems. This process builds artificial neural networks composed of interconnected layers, enabling breakthroughs in computer vision, natural language processing, and other fields. For instance, large language models like OpenAI’s GPT-4 learn autonomously by predicting text sequences—consuming trillions of words without direct human input.
After foundational training, these models engage in supervised fine-tuning, wherein human input shapes their output towards desirable responses. Additionally, techniques like reinforcement learning with human feedback help refine their decision-making boundaries.
What Are AI’s Strengths?
- AIs excel at language tasks and consistently outperform humans on standardized tests.
Kanan: AIs demonstrate exceptional proficiency in language-related tasks, including translation, essay writing, and editing. Notably, models like GPT-4 achieve remarkable scores on standardized assessments, ranking in the top percentiles for exams such as the Bar Exam (90th percentile) and the LSAT (88th percentile). Above all, they even surpass human performance in emotional intelligence, which is a surprising revelation.
Moreover, AI can act as a co-scientist—assisting researchers in generating hypotheses, drafting proposals, and synthesizing scientific literature. They’re becoming integral to multimodal systems that blend vision and language tasks, robotics, and decision-making.
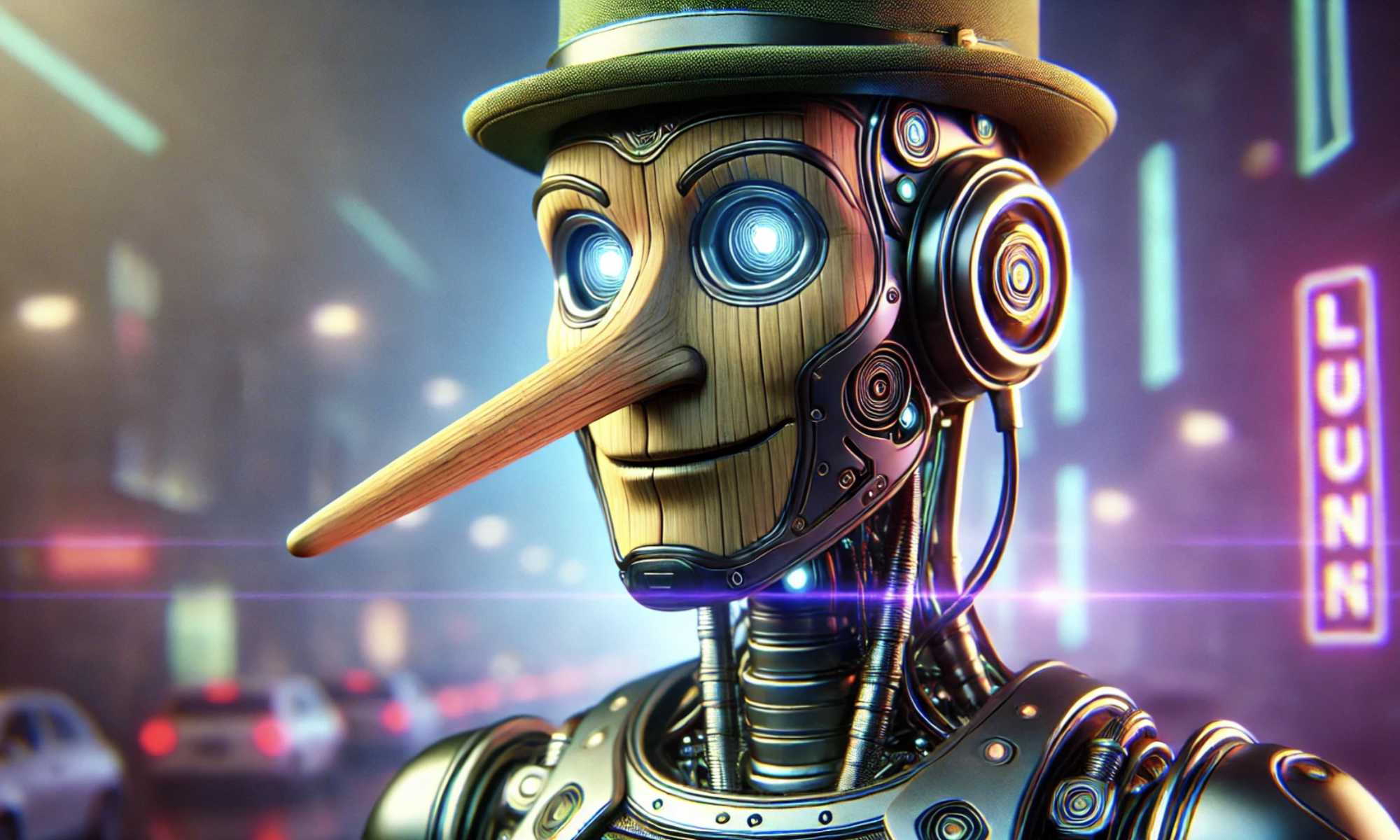
What Limitations Remain for Generative AI Tools?
- Generative AI systems currently lack self-awareness and sophisticated reasoning.
Kanan: Despite advancements, LLMs can still generate erroneous content, a phenomenon termed “hallucination.” Their reasoning and planning capabilities lag behind human proficiency, and these systems don’t continually learn, resulting in a static knowledge base post-training that lacks awareness of current events.
“Current generative AI systems also lack metacognition, which means they typically don’t know what they don’t know. This absence of self-awareness limits their effectiveness in real-world interactions.”
This inability to ask clarifying questions when faced with ambiguity curtails their applications. Human learners build on foundational skills, making progressive strides in increasingly complex capacities—something that LLMs fail to replicate effectively, a challenge my lab is devoted to solving.
What Challenges and Risks Does AI Bring?
- AI is transforming the workforce, sparking debates on regulation.
Kanan: The rise of generative AI is already revolutionizing industries, especially those with white-collar roles where AI tools can empower individuals to boost their productivity considerably. This shift raises questions about job security and the future of work, given the potential for widespread automation in many sectors.
“Concerns about regulation exist, particularly regarding the possibility that it could stifle innovation while also concentrating AI benefits among a select few.”
While dystopian scenarios like the infamous “Paperclip Maximizer”, where AGI unintentionally threatens humanity, dominate discussions, a more immediate peril stems from humans deliberately wielding AI for destructive ends. Emphasizing international collaboration and ethical AI development, we must seek to invest in safety research while promoting effective regulatory measures.
What Are the Chances of Achieving Artificial General Intelligence (AGI)?
- Many researchers firmly back the feasibility of AGI, yet current LLMs are too constrained.
Kanan: Renowned Turing Award winners—Yoshua Bengio, Geoffrey Hinton, and Yann LeCun—unanimously agree that AGI is achievable. However, caution is paramount, given potential existential risks associated with AGI advancements. Nonetheless, it remains widely agreed that the existing architecture of LLMs can’t realize AGI.
Crucially, LLM reasoning is tied to language, which constricts their ability to engage in abstract thought, whereas humans utilize language primarily as a communicative tool, not the framework for thought itself.
Meet Your Expert: Christopher Kanan
Christopher Kanan
Associate Professor of Computer Science
Christopher Kanan serves as an associate professor of computer science at the University of Rochester, holding secondary appointments in the Department of Brain and Cognitive Sciences, the Goergen Institute for Data Science, and the Center for Visual Science. He is a leading expert in artificial intelligence, continual learning, vision, and brain-inspired algorithms.